Structural dynamics of proteins:
Extracting the real-time biological function of molecular machines, membrane proteins, and enzymes is of significant interest in structural biology. This is now feasible with the advent of modern X-ray Free Electron Laser (XFEL) machines. Nevertheless, many algorithmic and data analysis challenges still remain. For example, with the substantial timing uncertainties and extreme noise and sparsity involved in the time series of X-ray diffraction data from crystallized proteins, it becomes very difficult to recover dynamics at high temporal resolutions. The customary techniques in biophysics are thereby constrained while their outcomes are usually at low time resolutions, making it very difficult to study interesting ultrafast structural dynamics that occur in few-femtosecond time intervals. Therefore, novel and powerful data-analytic approaches are required to deal with such timing inaccuracies. To this end, we have worked on the structural dynamics of a photoactive protein using time-resolved data taken by XFEL technology. By developing an advanced data-driven machine learning algorithm and with the help of quantum dynamical simulations, the chromophore isomerization of such a complex molecule is accessible with atomic spatial resolution and few-femtosecond time resolution, which is far better than traditional methods (see this article for more details). This approach can be applied to other photosensitive proteins.
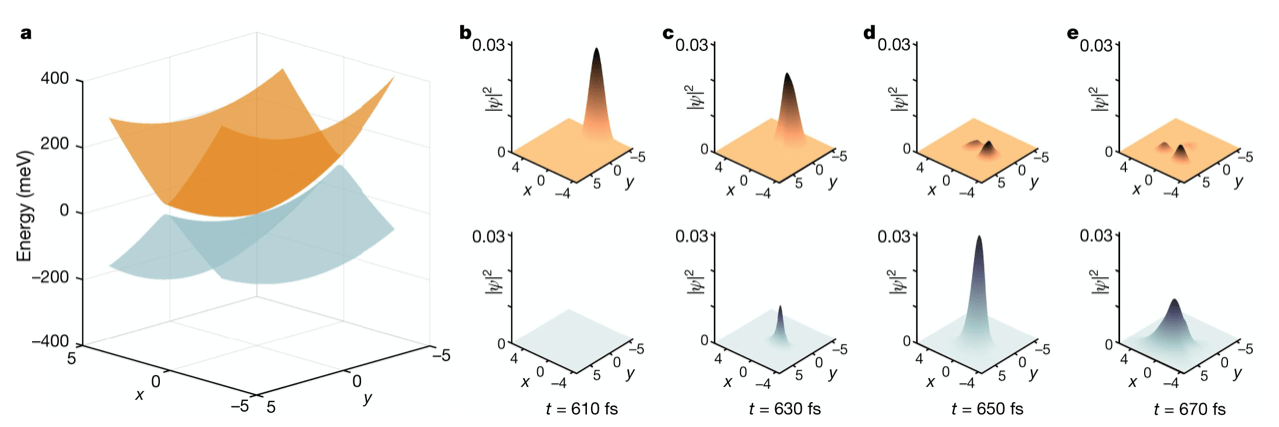
Topography of the conical intersection and the associated population dynamics in a photoactive protein (A. Hosseinizadeh et al, Nature 599, 2021)
Structure determination of biomolecules:
Finding high-resolution structures of biomolecules is a key step to obtaining biologically relevant information from these particles. Whether to employ electron microscopy or X-ray imaging techniques, algorithmic tools must be used to recover the structure of these objects from (noisy) snapshots. This, in turn, is essential for extracting real structural changes of these objects and compiling high-resolution 3D videos along functional paths on energy landscapes. For this goal, and in the context of X-ray imaging, we have developed a novel machine-learning algorithm capable of extracting high-resolution three-dimensional structures of icosahedral viruses from large ensembles (up to millions) of X-ray snapshots (see here).
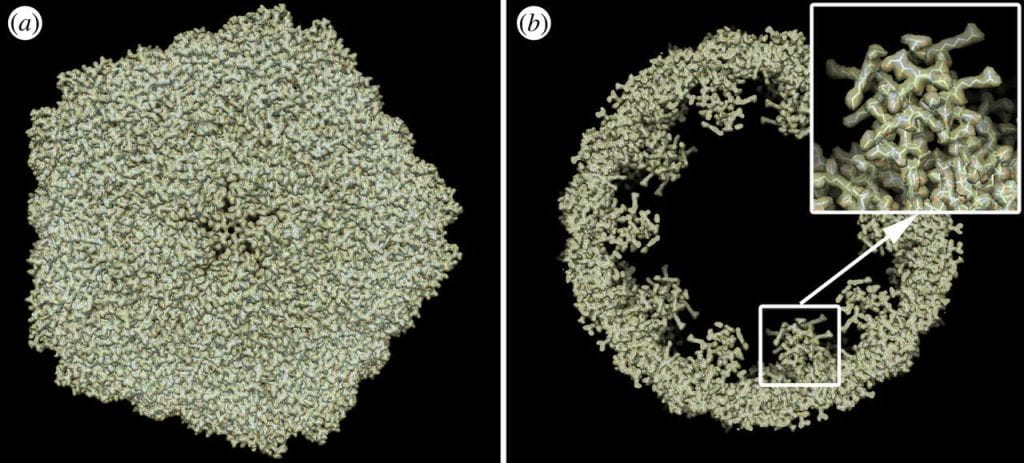
Atomic structure of a simulated icosahedral virus by machine learning (A. Hosseinizadeh et al, Phil Trans B 369, 2014)
Structural conformations:
The above-mentioned approach combined with a sophisticated non-linear singular value decomposition technique, not only creates 3D pictures of single viruses but also generates 3D videos capturing these particles in different conformational states along their functional pathways (see here). Besides, we can also retrieve the structural conformations and functions, as well as the thermodynamics of molecular machines using electron microscopy data (see this article).

Structural conformations of a virus from single-particle X-ray imaging (A. Hosseinizadeh et al, Nature Methods 14, 2017)
Data analytics:
Nowadays, there is no doubt that data science plays a pivotal role in modern science and technology. Deriving meaningful information from large collections of multi-dimensional data requires the development of advanced algorithms and computational methods. In addition to research in structural biophysics, we are generally interested in data analytics, particularly based on supervised and unsupervised machine learning approaches. In the past years, we have put a lot of effort into data classification, data cleaning, and purification, and data preprocessing tasks such as recognition and reduction of stochastic artifacts and anomalies, which are essential steps in data analysis to avoid incorrect results and misleading conclusions when mining the information content of data (for example, see this, this, and this papers).

Artifact removal in X-ray snapshots by machine learning (A. Hosseinizadeh et al, Structural Dynamics 2, 2015)
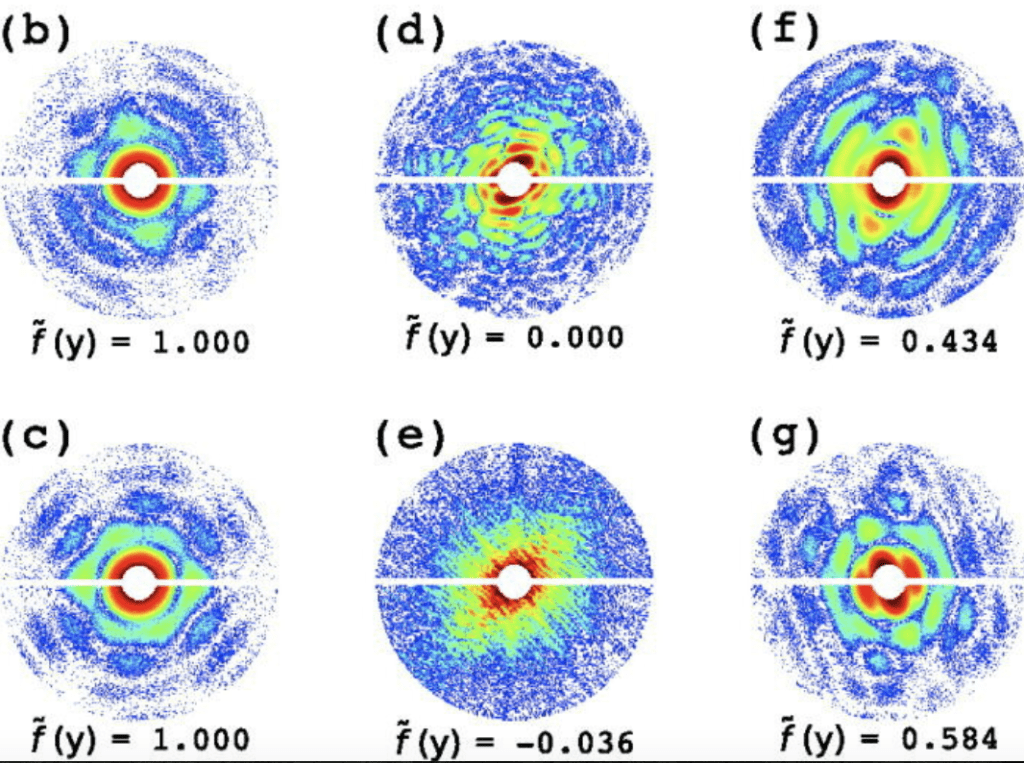
Data classification by supervised learning (E. Cruz-Chu et al, Structural Dynamics 8, 2021)
Ongoing and prospective projects:
- We are currently studying the femtosecond structural conformations and dynamics of phytochrome proteins using time-resolved X-ray crystallography data. This study is of particular interest because phytochrome photoreceptor proteins play a crucial role in the development and growth of plants on Earth (see this reference for more information).
- The compact X-ray free electron laser (CXFEL) is a 5-year term project being developed at Arizona State University. It is designed to be a more compact and accessible version of the traditional large-scale and expensive XFEL facilities. We are developing intelligent and optimized data collection and analysis packages using machine-learning methods for this project. The CXFEL will have a wide range of applications in structural biology, material science, fundamental physics, and chemistry.
- The new generations of XFEL instruments with high repetition rates are able to collect up to millions of snapshots in a single experiment. Enhancing the existing computational resources and algorithms or developing innovative approaches capable of reducing large masses of data into manageable parts together with useful information retrieval are of significant importance. Despite a lot of progress in this direction, there is more room for developing new methods or the improvement of existing ones, and this is our other research perspective.
- Apart from structural biophysics with X-ray lasers and electron microscopes, the data-analytic methodologies that we have been working on are also extendable to other territories of science, engineering, and medicine. This in turn indicates a remarkable capacity for building new collaborations that we continuously take into consideration.
Join us!